Machine Learning Operations, also known as MLOps refers to a set of practices that standardizes end-to-end Machine Learning processes. This includes gathering data for the Machine Learning to monitoring it in production, and everything in between. In this blog post, I will talk in brief about MLOps, a skillset that is going to be in high demand in the years to come.
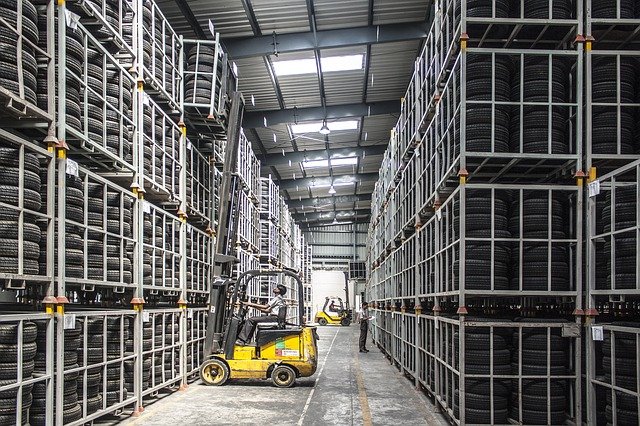
Machine Learning has been a field of active research for years now. However, only recently has there been widespread adoption of Machine Learning into the industry. But this widespread adoption has resulted in its own set of problems and hurdles. This problem arose out of the non-standard practices being followed to train and maintain Machine Learning. Consequently, standardization of Machine Learning processes is the need of the hour. Thankfully, this is not the first time that the software industry has faced this kind of issue with standardization. In the past few decades, the process of standardizing Software Development and its Operations by using DevOps methodology has been a wild success. Nowadays, similar practices are being applied for the development and maintenance of Machine Learning models. These practices are called MLOps, named after their software counterparts DevOps.
Machine Learning is driving value and opening up a world of opportunities for businesses unlike anything has ever done before. This world of opportunities, however, is fraught with challenges. As businesses are starting to leverage Machine Learning models, they realize the importance of keeping the model operational and relevant. And this is where MLOps comes in. As more and more industries and businesses start to leverage Machine Learning to drive the value of their business, it is going to become important to standardize the process of developing, maintaining, and monitoring the development of ML models.
For these reasons, it becomes important for ML Practitioners and Data Scientists to acquire the very important skills related to MLOps. This includes not just the tools, technologies, and software that will be used for practical MLOps but also other important skills that will be complementary to it such as Statistics, the MLOps feedback loop, etc.
The process of MLOps begins even before the data collection has been performed and continues well after the model is deployed. This is different from the conventionally used one-off deployments where the model is trained and moved into production, and that is it. The model is not monitored and improved upon. By applying the practices of MLOps, best practices are employed right from the start. There is an emphasis on practices like versioning, proper documentation, and paper trail, responsible and explainable AI, and repeatability. Additionally, there is also an emphasis on continuous automated monitoring of the model, and in case, if required, automated or semi-automated(only the final decision is taken manually) retraining of the model.
The benefits of MLOps are also huge. Due to the automation of many tasks, Data Scientists can focus on more important and pressing problems, such as trying out novel models and ideas instead of manually performing tasks that can be easily automated. This leads to an increase in the work satisfaction of the Data Scientists, making them more likely to stay in the organization. Automating tasks also benefits the organization from a cost standpoint, as the data science talent, which is already very expensive and still difficult to get, will be used for more purposeful tasks trying to solve new business instead of maintaining the old systems.
Furthermore, MLOps can be beneficial from a regulatory standpoint as well. Repeatability, Versioning, and Documentation will be required from complying with guidelines by the regulatory authorities for ensuring the Artificial Intelligence/ Machine Learning systems used are fair, explainable, and unbiased. MLOps will also benefit the organization in case of any unforeseen events, such as loss of Human Resources, and also onboarding new Human Resources to the team.
All these factors will create a rise in the demand for people who are well versed and know how to apply MLOps skills to a project. Many Machine Learning ecosystems such as Tensorflow are extending the ecosystem to include MLOps functionalities by creating additional libraries such as Tensorflow Extended(or TFX). The trajectory for the future of MLOps is clear: The use of MLOps and the demand for MLOps talent will increase in the future.