The field of Artificial Intelligence, and particularly Computer Vision has witnessed a lot of advances in recent years. Prior computer vision fields such as image classification have seen appreciable improvements in classification accuracy. Whereas various new fields and use cases have never ceased to amaze us. New neural network architectures, use cases, applications, and training methods have also contributed a lot to the rapidly changing field. In this blog, we will take a look at the top 3 Computer Vision trends which you should be looking out for this year. These fields have already attracted a lot of attention in the past years and are all set to amaze us, even more, this year.
Neural Radiance Fields
Neural Radiance Fields, also known as NeRF first came out in March 2020 in a paper with the title “NeRF: Representing Scenes as Neural Radiance Fields for View Synthesis”. The paper was authored by researchers from UC Berkeley and Google Research. It was capable of rendering new views of complex geometric objects from a set of input views. NeRF was able to achieve state of the art results in view synthesis by optimizing an underlying continuous volumetric scene function. NeRF however has its own disadvantages, especially with variable lighting conditions and obstructions. These shortcomings were improved by NeRF-W(NeRF in the wild). Deformable Neural Radiance Fields, also known as D-NeRF is another new NeRF technique. It is used to convert images or videos that are captured casually using a mobile phone to produce photorealistic renderings of the subject from arbitrary viewpoints. These rendering a called “nerfies”.
NeRF is a new topic in computer vision but it is already showing amazing results. It has even managed to attract a lot of attention in such a short amount of time. This makes NeRF one of the newest entrant into computer vision trends.
Super Resolution
Super Resolution is a field of Computer Vision that concerns with converting a low resolution image/video into a high resolution one. It has been a hot topic in computer vision for quite some time now. In recent years, the use of techniques such as TecoGAN has been quite successful and delivered very promising results. Every year we see tremendous improvement in this field, and hopefully, this year too will bring a lot of improvements in the field of Super Resolution.
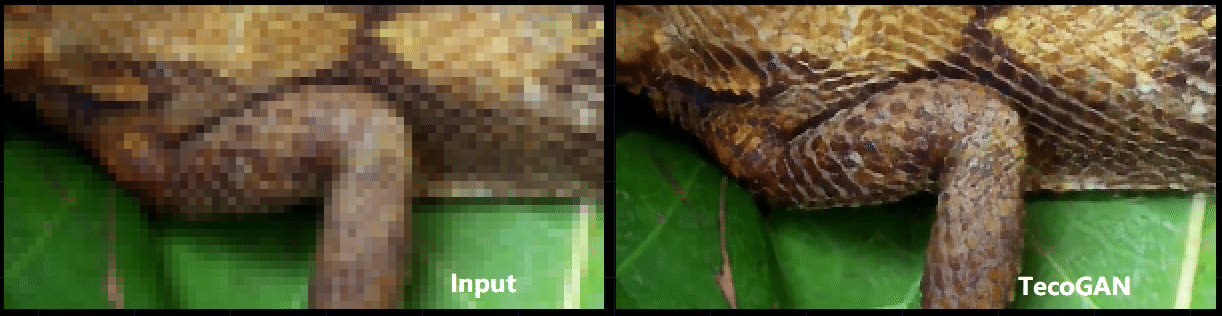
We are really excited about further improvements coming in the field of super resolution this year.
Video Inpainting
Image Inpainting is the task of reconstructing missing regions in an image. This technique can also be used to remove objects from the image, see Fig. 1. Image Impainting has been here for quite some time now and has achieved amazing results.
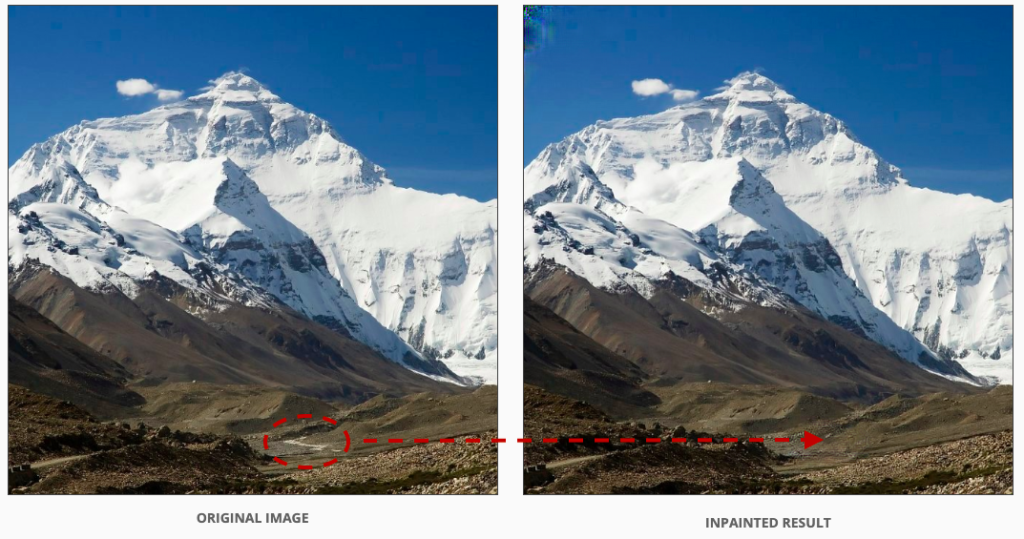
However, in the last few years, there has been considerable improvement in Video Inpainting too. It is the task of removing objects from the video by reconstructing the video after its removal. As if this in itself was not enough, video inpainting techniques are also capable of removing reflections and shadows of the objects from the video. In one of the video inpainting techniques[Gao et al, 2020] it is even possible to perform a spatial expansion of the video.
With the latest video inpainting techniques, it is hard to even tell if the object ever existed in the first place. Video inpainting is one of the most amazing trends to look out for in the field of Computer Vision in the coming years.