Microsoft Research, the research subsidiary of Microsoft has announced AI4Science. AI4Science will consist of a global team of machine learning, quantum physics, computational chemistry, molecular biology, fluid dynamics, and software engineering experts working to tackle important societal challenges.
Microsoft Research announces AI4Science, a new global team of machine learning, quantum physics, computational chemistry, molecular biology, fluid dynamics, and software engineering experts working to tackle important societal challenges. Learn more: https://t.co/4hZhxKrhUZ
— Microsoft Research (@MSFTResearch) July 7, 2022
The use of Artificial Intelligence and Deep Learning in Natural Sciences can have a transformative impact on natural sciences with far-reaching consequences. One such consequence of AI in Natural Sciences would be in the field of medicine and drug discovery. For example, the number of small-molecule drug candidates alone is estimated at 1060, while the total number of stable materials is thought to be around 10180
As a matter of fact, the AI4Science team at Microsoft has used the platform to generate several promising early-stage molecules which have been synthesized for further exploration.
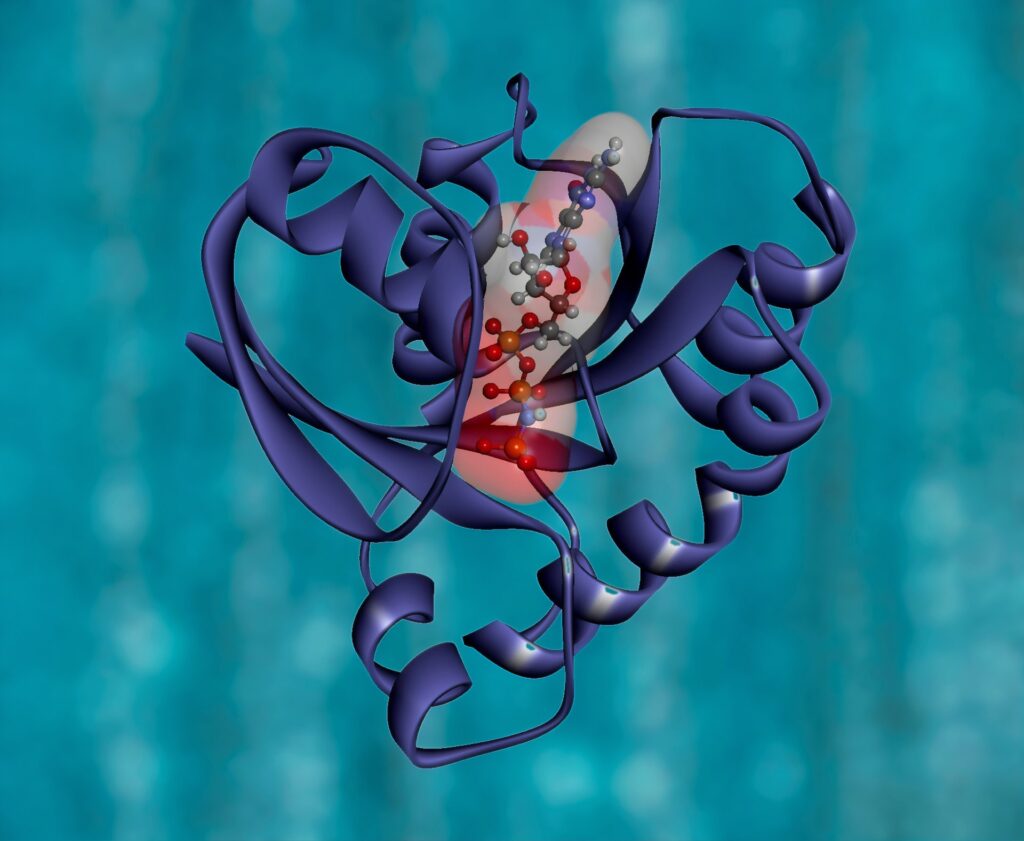
“Over the coming decade, deep learning looks set to have a transformational impact on the natural sciences. The consequences are potentially far-reaching and could dramatically improve our ability to model and predict natural phenomena over widely varying scales of space and time. Our AI4Science team encompasses world experts in machine learning, quantum physics, computational chemistry, molecular biology, fluid dynamics, software engineering, and other disciplines, who are working together to tackle some of the most pressing challenges in this field.“
Professor Chris Bishop, Technical Fellow, and Director, AI4Science
Combining Deep Learning with other emerging fields of technology such as Quantum Computing will put Deep Learning on Steroids. One application of Quantum Computing in the field of Chemistry and Medicine is the simulation of molecules, which is quite impossible for Classical Computers to do for even small molecules.
Further, Quantum Computing also has applications in speeding up Machine Learning algorithms.
AI4Science is an effort deeply rooted in Microsoft’s mission, applying the full breadth of our AI capabilities to develop new tools for scientific discovery so that we and others in the scientific community can confront some of humanity’s most important challenges. Microsoft Research has a 30+ year legacy of curiosity and discovery, and I believe that the AI4Science team – spanning geographies and scientific fields – has the potential to yield extraordinary contributions to that legacy.
Kevin Scott, Executive Vice President and Chief Technology Officer, Microsoft
Currently, the AI4Science team is based in Amsterdam, Beijing, Cambridge, and Shanghai.
Paradigms of Scientific Discovery
Jim Gray, a Turing Award winner, and former Microsoft Technical Fellow, characterised the historical evolution of scientific discovery through four paradigms.
“With origins dating back thousands of years, the first paradigm was purely empirical and based on direct observation of natural phenomena. While many regularities were apparent in these observations, there was no systematic way to capture or express them”. “The second paradigm was characterised by theoretical models of nature, such as Newton’s laws of motion in the seventeenth century, or Maxwell’s equations of electrodynamics in the nineteenth century. Derived by induction from empirical observation, such equations allowed generalization to a much broader range of situations than those observed directly”.
“While these equations could be solved analytically for simple scenarios, it was not until the development of digital computers in the twentieth century that they could be solved in more general cases, leading to a third paradigm based on numerical computation”. “By the dawn of the twenty-first century computation was again transforming science, this time through the ability to collect, store and process large volumes of data, leading to the fourth paradigm of data-intensive scientific discovery. Machine learning forms an increasingly important component of the fourth paradigm, allowing the modelling and analysis of large volumes of experimental scientific data”.
These four paradigms are complementary and coexist.
“However, over the last year or two, we have seen the emergence of a new way to exploit deep learning, as a powerful tool to address this speed-versus-accuracy trade-off for scientific discovery”. “This is a very different use of machine learning from the modelling of data that characterizes the fourth paradigm, because the data that is used to train the neural networks itself comes from numerical solution of the fundamental equations of science rather than from empirical observation”.
Learn more about the Microsoft AI4Science team here.
See career opportunities at Microsoft AI4Science here.
Other Articles you might be Interested In
Ian Goodfellow, the inventor of Generative Adversarial Networks moves to DeepMind
First Edition of IEEE Conference on Secure and Trustworthy Machine Learning scheduled for 2023
YouTube Launches Its Research Program
Alphabet spins out SandBox AQ, an AI and Quantum Computing Company